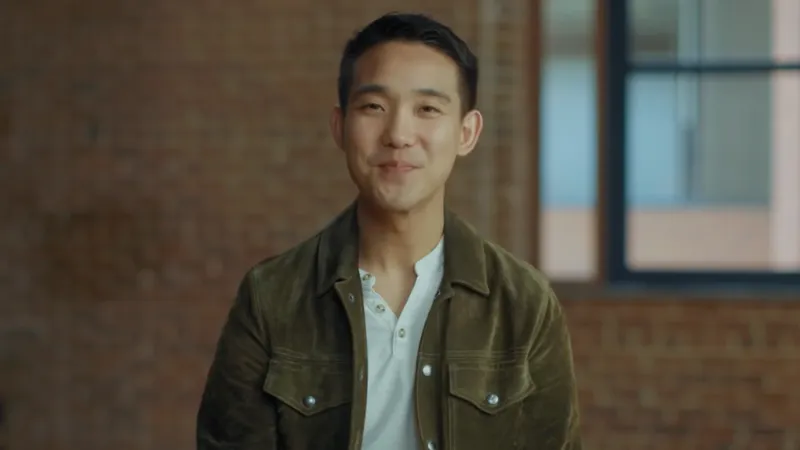
Goodfire, a cutting-edge AI research company focused on making neural networks understandable and controllable, has raised $50 million in a Series A funding round led by Menlo Ventures. The round also saw participation from Lightspeed Venture Partners, Anthropic, B Capital, Work-Bench, Wing, South Park Commons, and others. The funding arrives less than a year after the companyās founding.
Also read ā 1Fort Raises $7.5M to Transform Business Insurance with AI
The capital will accelerate Goodfireās interpretability research and support the development of its flagship platform, Ember, which offers deep, programmable access to a modelās inner workingsāmoving beyond black-box behavior to enable better training, alignment, and performance.
With this funding, Goodfire plans to expand its team, strengthen research collaborations with frontier AI developers, and continue pushing the boundaries of model interpretability in domains like image processing, scientific modeling, and advanced language reasoning.
Ember allows users to unlock hidden knowledge, reshape model behavior, and gain unprecedented insight into model decisions. The platform has already shown results in scientific fields, helping early partner Arc Institute gain breakthroughs in biological research using Evo 2, its DNA foundation model.
āNobody understands the mechanisms by which AI models fail, so no one knows how to fix them,ā said Eric Ho, Co-founder and CEO of Goodfire. āWeāre building tools to change thatāfrom the inside out.ā
Also read ā Exaforce Raises $75M in Series A Round Funding
āGoodfire is cracking open the AI black box,ā said Deedy Das, investor at Menlo Ventures. āTheir technology will redefine how enterprises build and deploy trustworthy AI systems.ā
Anthropic CEO Dario Amodei added, āMechanistic interpretability is a critical foundation for responsible AI development. Goodfire is leading the charge.ā
About Goodfire
Founded by a team of researchers from OpenAI and Google DeepMind, Goodfire is pioneering mechanistic interpretability, a science that decodes how neural networks operate internally.