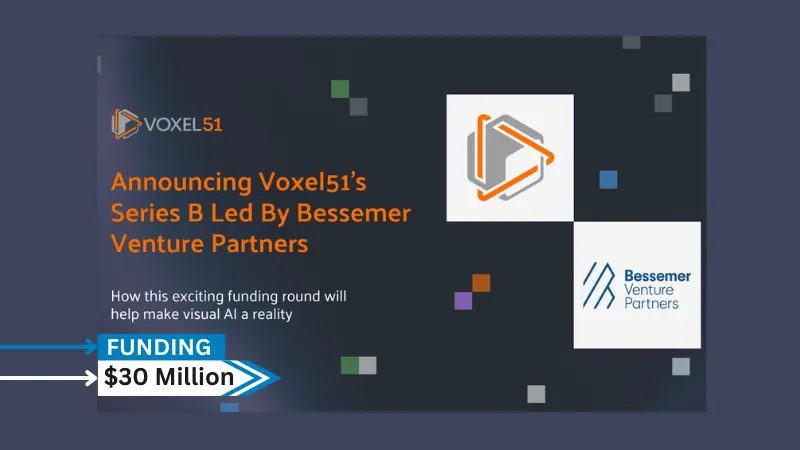
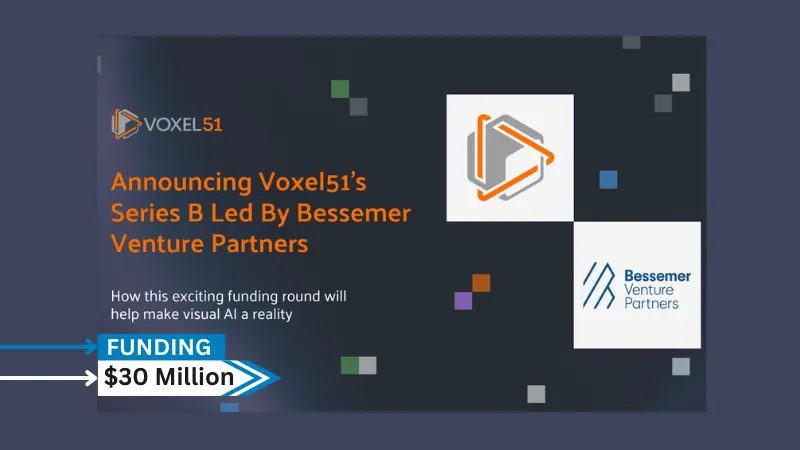
Voxel51, a company which specializes in visual AI secures $30million in series B round funding. This round was led by Bessemer Venture Partners, with participation from new investor Tru Arrow Partners and existing investors Drive Capital, Top Harvest Capital, Shasta Ventures, and ID Ventures.
This milestone marks an exciting inflection point in their growth at Voxel51, and it comes at the cusp of an AI-driven revolution. It’s clear that AI is here to stay, fueled by a unique mixture of simultaneous advancements in hardware, algorithms, and an explosion of new use cases that stimulate the imagination and, importantly, are causing organizations to think deeply about how to unlock the value in their massive stores of unstructured data through AI-powered products and services.
At Voxel51, they were especially excited about the rapid progress of visual AI. Image and video data is everywhere, making up a majority of all data traffic. Use cases from defect detection, to augmented reality, to autonomy are reaching production every day. Advancements in generative AI bring promises of personalized product experiences and solutions for roadblocks like data diversity and the prohibitive cost of human annotation.
Read also – [Funding News] NYC-based Cover Genius Secures $80Million in Series E Round Funding
Visual data is a critical input to solving society’s most challenging problems, and visual AI provides the reasoning that will close the loop on general intelligence systems that will solve them.
Voxel51’s products help their customers and users build dramatically better AI systems by bringing their models and data together in one place where they can orchestrate and automate workflows for examining, curating, evaluating, and iterating on their data and models, together. They provide the connective tissue that brings harmony to their AI processes and pushes projects into production.
About Voxel51
Voxel51 is an AI software company that enables machine learning and computer vision scientists to rapidly curate and experiment with their datasets in order to build higher performing machine learning systems.